How to Prepare Your Team for AI Implementation: A Practical Roadmap
Industries are rapidly evolving with AI. Ready your teams with targeted upskilling, stakeholder support, and a culture of innovation. This concise guide covers skill gaps, ROI, and how to thrive in an AI-driven future.
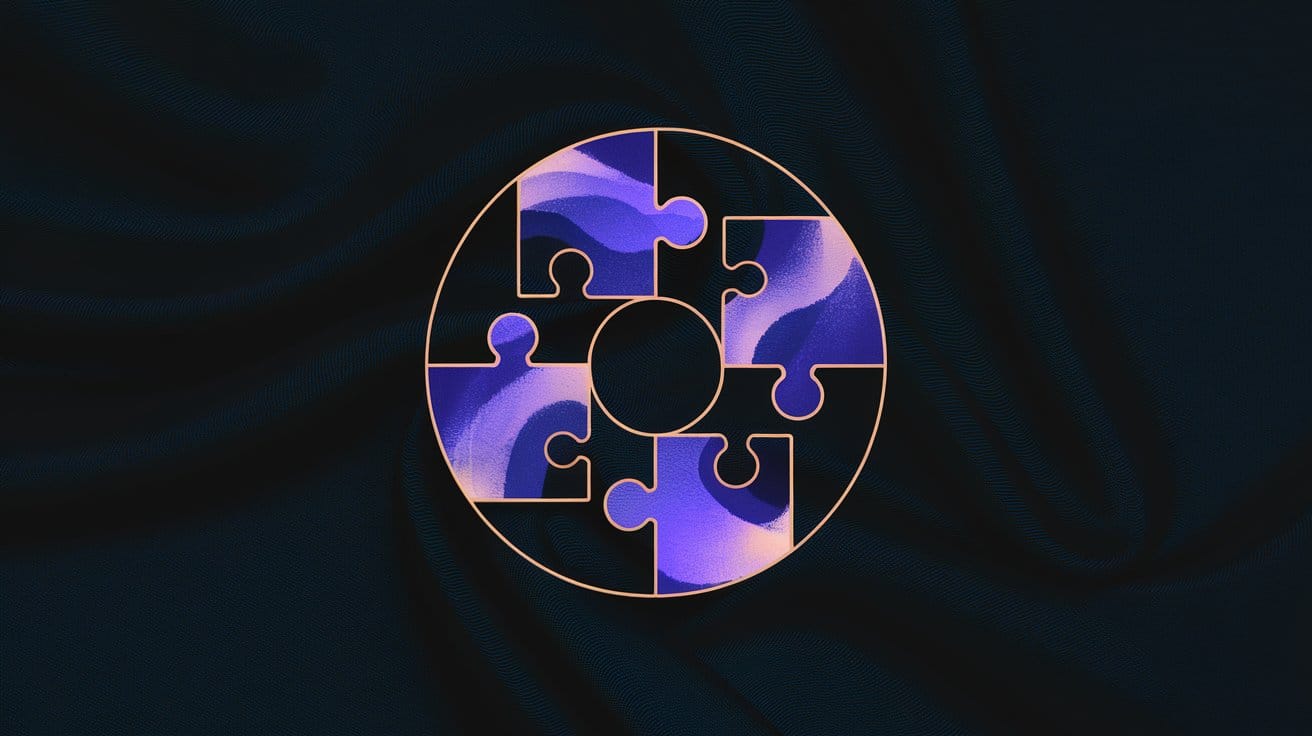
Have you noticed how AI is racing ahead, transforming industries in the blink of an eye? According to recent research on AI maturity, organizations everywhere are scrambling to catch up. This guide is here to help you skill up your teams—focusing on which roles need AI know-how, how to approach training, what cultural shifts matter most, and how to measure success. It’s aimed at leaders, HR directors, L&D professionals, and anyone else planning to equip their people with the AI skills needed to stay competitive. If you want to future-proof your workforce, this is for you.
By the end, you’ll have a roadmap to build an AI-savvy workforce that’s ready to compete and grow.
Main Challenges and How This Guide Addresses Them
- Identifying Skill Gaps
Discover practical ways to audit current capabilities and choose the right training methods. - Securing Buy-In
Learn to align executives, finance teams, and employees around a clear AI vision. - Budget & ROI Concerns
Get tips on keeping costs under control and showing a return on your AI investments. - Cultural Shifts
Build morale by explaining AI’s value and spotlighting new employee opportunities.
Follow these steps, and you’ll be well on your way to nurturing an AI-powered organization.
Why Upskilling in AI Matters
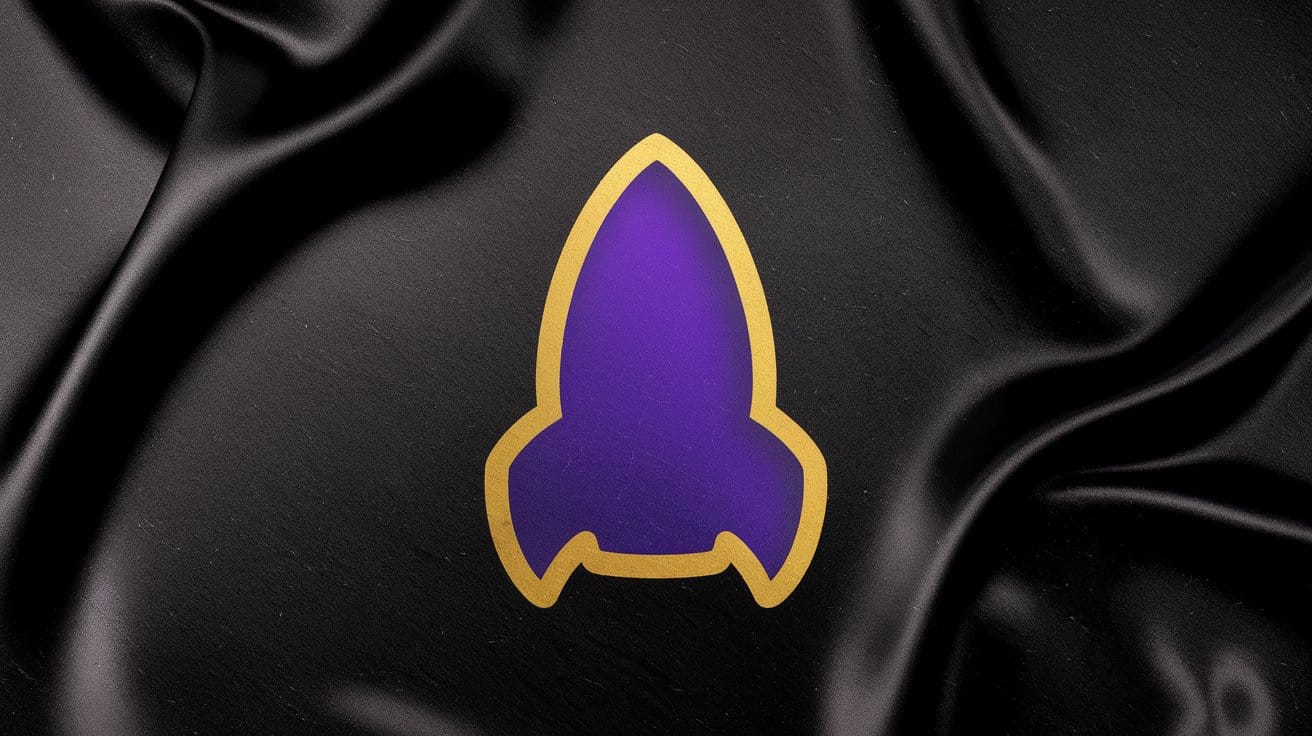
The Importance of AI in Modern Organizations
AI isn’t just a buzzword for tech teams anymore. It’s influencing everything—marketing strategies, customer interactions, finance workflows, and more. From automating routine tasks to providing predictive insights and natural language interfaces, AI opens doors to more efficient, creative, and value-packed outcomes. A workforce that’s comfortable with AI can instantly interpret data, personalize customer experiences, and eliminate unnecessary busywork. In short, they can focus on bigger, more strategic contributions (check out these real-world success stories).
Risks of Not Having an AI-Skilled Workforce
Ignore AI, and you risk watching more adaptable, data-driven competitors leave you behind. Employees who lack up-to-date AI skills can start feeling lost or alienated, with morale issues becoming a real concern (AI-related frustration is rising). If your skill sets never evolve, you’ll see turnover climb, enthusiasm dip, and growth opportunities shrink. In a world where innovation speeds up by the day, teams who aren’t prepared for AI face a tough road ahead.
Understanding Organizational Readiness
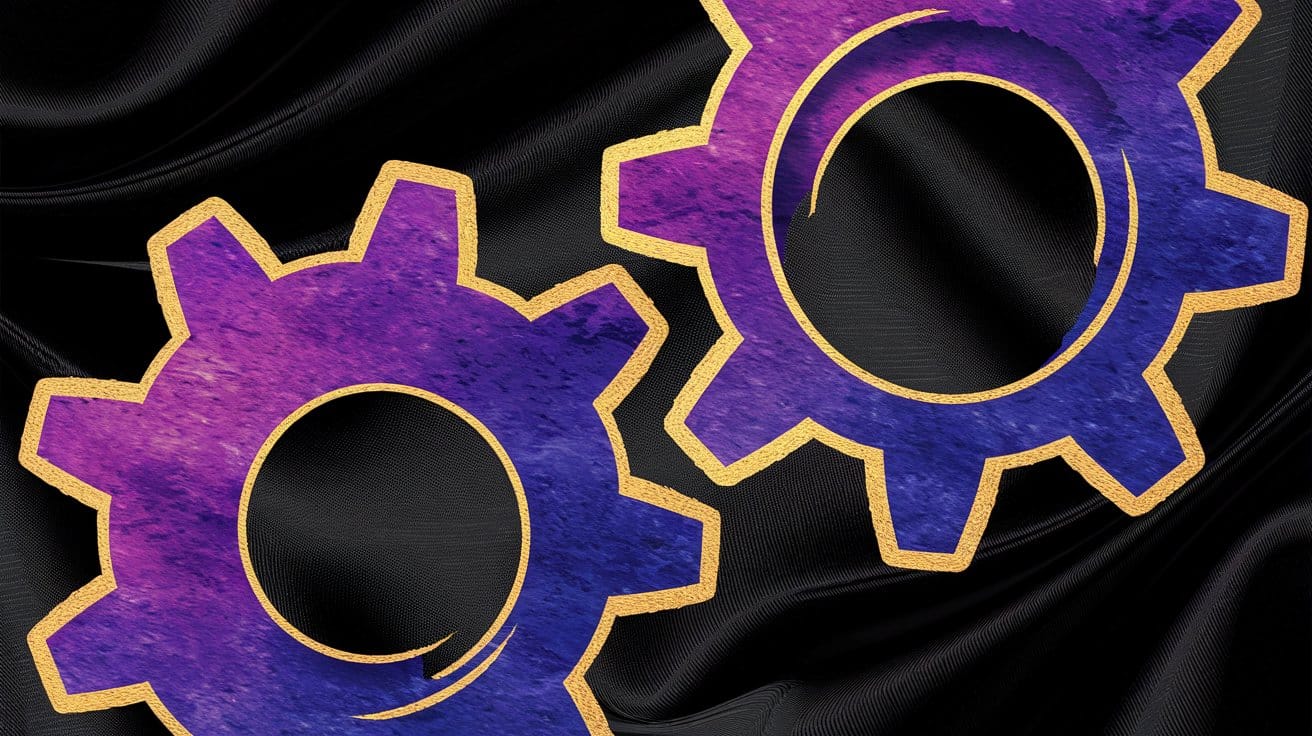
Assessing Current Skill Sets and Infrastructure
Before you dive into training, it pays to take a close look at your existing setups and people:
- Data Management: Are your data pipelines clean, consistent, and centralized?
- Technical Skills: Who’s already familiar with Python, statistics, or data analysis tools?
- Business Context: Which departments need basic AI literacy, and which need advanced skills?
Mapping out these capabilities helps you target the biggest impact areas first.
Identifying Myths and Misconceptions
Many still worry AI will make human roles obsolete or that only the IT team should care. In reality, AI often augments roles, freeing individuals to focus on strategy and innovation instead of mind-numbing tasks. It makes people more efficient. Address these fears head-on so everyone stays aligned and open to learning.
Growing Organizational Pressures and Opportunities
Digital transformation is picking up steam. AI can tackle rising customer demands, open up new revenue streams, and foster deeper analytical skills. Meanwhile, AI talent is hotly sought after. Training your people internally not only retains their company-specific knowledge but also cultivates a forward-thinking culture that keeps pace with industry demands.
Building an AI Upskilling Roadmap
Defining End Goals
Start with crystal-clear targets: maybe “Cut down manual data entry by half” or “Adopt AI-driven inventory forecasting within six months.” These specifics guide your curriculum and justify your budget. Keep a concise “AI-readiness statement” so everyone knows where you’re heading.
Aligning Stakeholders and Securing Buy-In
- Executives: Present the ROI, competitive advantages, and potential cost savings.
- Finance Teams: Show how investments will pay off in both the short and long run.
- Employees: Highlight how new skills can boost job security and career paths.
Consider launching cross-departmental “AI advocacy groups” to champion these goals and calm any concerns early (leadership support).
Budget, Resource Allocation, and Timelines
You’ll need to plan for training platforms, instructor fees, software licenses, and employee time. If budget is a sticking point, focus first on the training that brings the quickest wins—like automating small but time-consuming tasks. Then build up to bigger transformations, like advanced analytics.
Defining Success Metrics
Track progress with both numbers and narratives:
- Time-to-Competency: How fast people master AI fundamentals.
- Error Reduction: Is data-entry or forecasting accuracy improving?
- Tool Adoption Rate: Are teams actually using the new AI tools?
- Productivity Gains: Is the overall workflow faster or more efficient?
Tailored Training Approaches
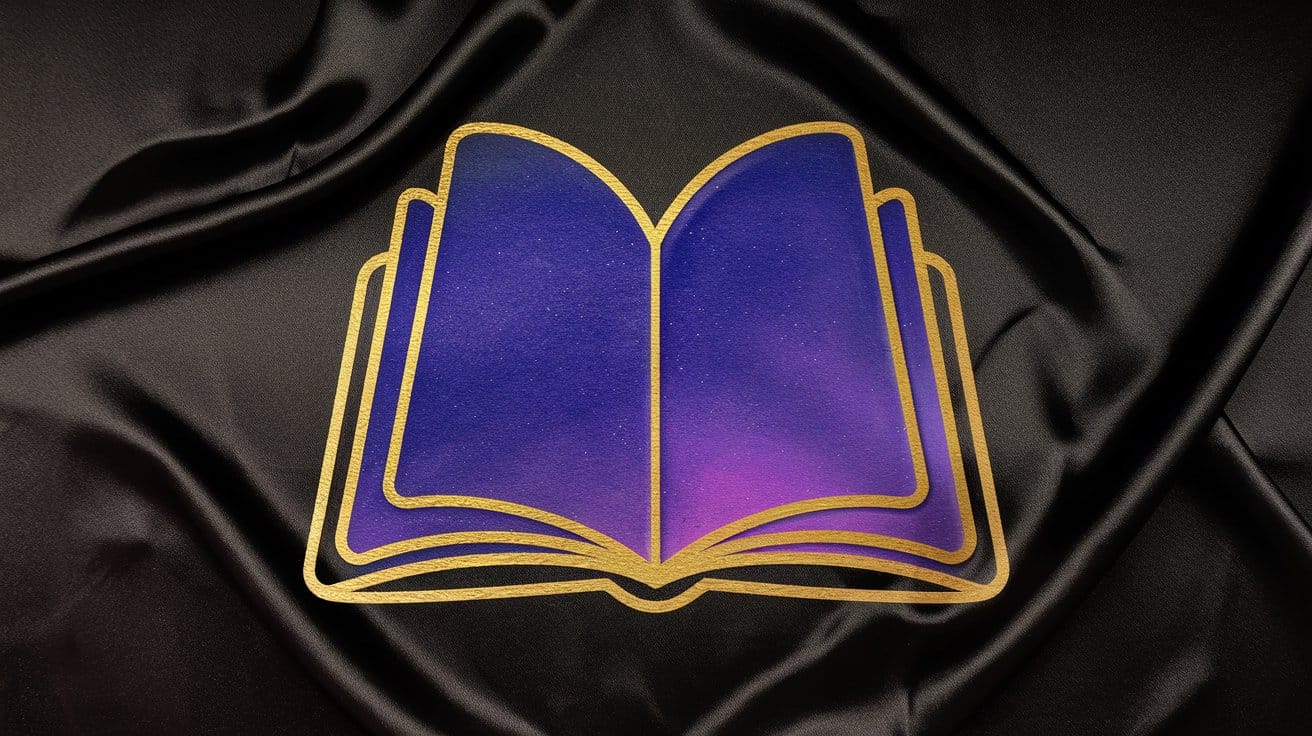
In-House vs. Third-Party Training
- In-House: Highly customized to your goals, and potentially cheaper over time, but you’ll need internal experts ready to train.
- Outsourced: Brings in specialized knowledge fast, though it may be more expensive and less tailored to your unique context.
Often, a mix of in-house and external sessions is best—covering your specific use cases in-house and letting outside pros handle more advanced topics.
Online Platforms and Microlearning
Platforms like Coursera and edX let team members learn at their own pace, from basic AI concepts to hands-on coding. Microlearning—short, bite-sized lessons—keeps motivation high, especially for those who don’t come from a tech background. Udemy is also another option, especially for more targeted courses on common AI tools like ChatGPT.
Workshops, Boot Camps, and Peer Learning
Want tangible prototypes and real demos? Host workshops or boot camps. Peer-to-peer “lunch and learns” can spark continuous improvement. When employees showcase their AI successes, it keeps morale high and encourages others to jump on board.
Role Identification and Team Structure
Critical Roles Needed
- Data Engineers: Ensure data is properly collected, cleaned, and integrated.
- Prompt Engineers and Prompt Architects: Design and optimize AI system interactions, serving as crucial liaisons between domain experts and AI systems. Architect complex prompt strategies to translate business requirements into precise, consistent, and high-value AI outputs.
- AI Specialists/ML Engineers: Develop machine learning models and pipelines.
- Domain Experts: Provide essential context for real business challenges.
- Business Strategists: Tie AI initiatives to broader organizational goals.
- Data Analysts/Citizen Data Scientists: Translate insights into actions and help promote AI solutions internally.
Figure out which experts you can realistically hire versus train up from within. Specialized roles often come with steep hiring battles, so cultivating homegrown talent can be a smart alternative.
Creating Cross-Functional Collaboration
AI thrives on the blend of tech, analysis, and business sense. Cross-functional teams keep projects rooted in actual needs, not “data science for data science’s sake.” Regular check-ins between departments fuel idea-sharing and help refine models continuously.
Evolving Job Descriptions
Expect roles to shift as AI matures. Marketers might dig into machine learning for hyper-personalization, while finance teams explore predictive analytics for smarter budgeting. Update job descriptions to reflect these new responsibilities—this transparency shows employees how they can grow with AI.
Establishing an AI-Ready Culture
Handling Resistance and Fears
Encourage open conversations to dispel the myth that AI equals job loss. Make it clear: AI usually offloads repetitive tasks, allowing people to tackle more meaningful work. Regular Q&A sessions help calm nerves and spark interest.
Encouraging Experimentation
It helps to create “AI safe zones,” where people can test ideas without fear of harsh consequences. Leaders who openly share how they’ve stumbled—and learned—show that experimentation is welcomed.
Leadership Role Modeling
When high-level executives adopt AI in their own decisions—like using data-driven dashboards for strategy—everyone else notices. It’s a powerful cue that AI is a critical piece of the company’s future.
Ethical and Risk Management Frameworks
Make ethics a priority from day one. Teach employees to spot bias, safeguard data privacy, and follow relevant AI regulations. Structured governance frameworks (e.g., IBM’s AI Governance) help ensure fairness and transparency, building trust both inside and outside your organization. Regularly audit AI systems for potential bias, and make sure there’s accountability in place for decisions aided by machine learning.
Practical Training and Implementation Steps
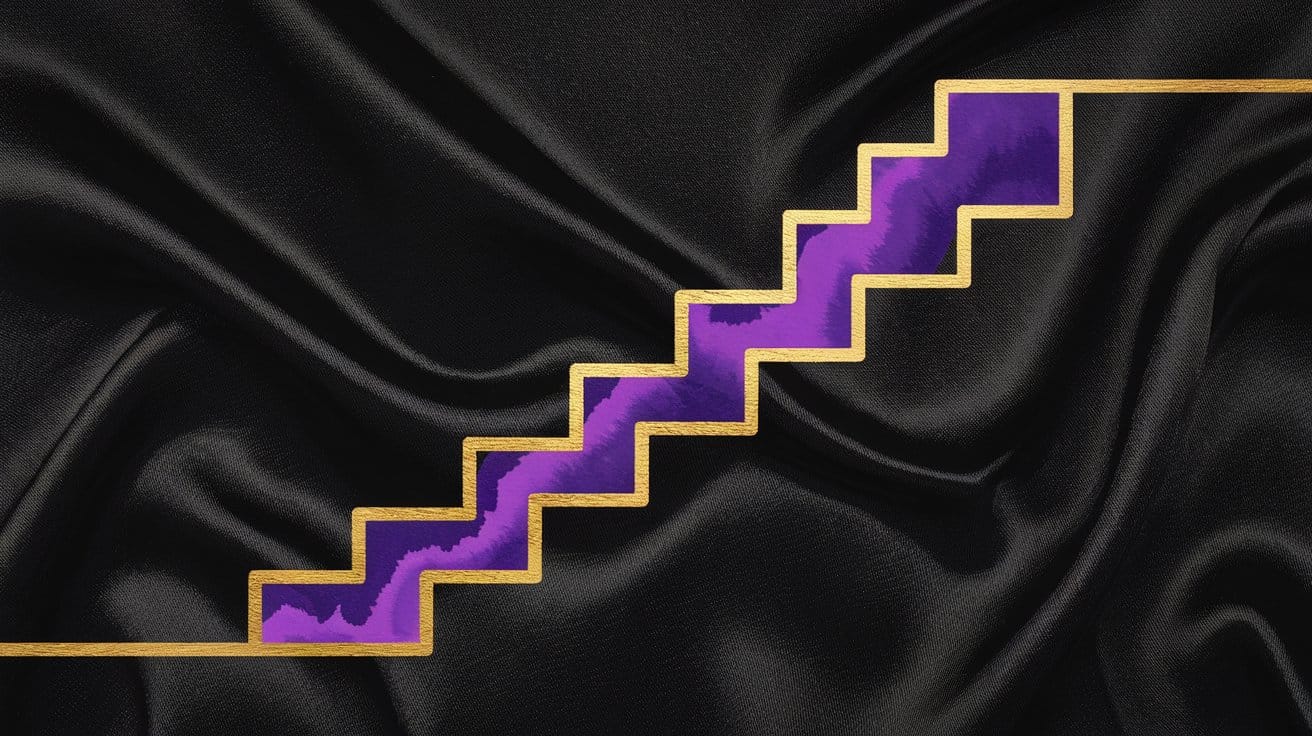
Phased Learning Model
- Intro to AI: Cover essential terms and basic concepts (supervised vs. unsupervised learning).
- Intermediate Labs: Get hands-on with mini-projects that solve actual departmental issues.
- Advanced Tracks: Provide deeper training for specialized roles—like machine learning engineering, prompt engineering, or large-scale data architecture.
This tiered approach keeps people from feeling overwhelmed and helps you spot rising stars who might specialize further.
Embedding Training into Daily Workflows
Apply skills immediately. Maybe a sales manager uses AI tools to predict churn, or a finance team member experiments with a machine learning model for budgeting forecasts. Recognize these early achievements to keep that forward momentum.
Pilots and Real-World Projects
Begin with smaller, manageable use cases: automating a monthly report or rolling out an AI chatbot for customer support. Measure the gains (in time, money, or satisfaction), learn from any hiccups, then broaden the scope. Early wins justify further investment and rally the team.
Measuring Impact and ROI
Quantitative Metrics
Track productivity spikes, cost savings, speed improvements, and how quickly new AI features reach the market. Industry frameworks (check out this guide here) offer more detail on how best to measure these.
Qualitative Indicators
Surveys and one-on-one chats can reveal how AI affects teamwork and collaboration. Notice whether people start seeking data-driven solutions on their own—that’s a clue you’re building a truly AI-driven culture.
Cost-Benefit Analysis and ROI Timelines
Balance short-term payoffs (like quick deployment of chatbots) with longer-term goals (like a full-blown AI transformation). Measure hard returns (e.g., revenue boost, expense cut) and softer benefits (like higher employee retention and better customer satisfaction).
Scaling and Continuous Improvement
Iterative Refinement of Training Programs
After every training session or module, gather feedback. Was it too advanced or too basic? Are employees using new skills well? Keep an eye on emerging trends like generative AI or evolving data privacy rules, and adapt accordingly.
Sustainable Knowledge Management
Capture your lessons and best practices in a shared “AI playbook.” Encourage code sharing, collaborative documents, and version control for data pipelines. That way, expertise endures even if certain team members move on.
Establishing a Post-Training Community of Practice
Set up a space—whether a physical room or an online forum—where people swap ideas, ask questions, and troubleshoot AI problems together. These communities keep AI momentum strong and help your organization mature its AI capabilities organically over time.
Future-Proofing Against Rapid AI Evolution
Put systems in place to regularly assess new AI breakthroughs or changes in regulation. That might mean adding advanced courses for topics like reinforcement learning or forming committees to evaluate ethical AI usage. By proactively scanning the horizon, you stay one step ahead of the rapid changes in this space.
Leveraging Existing AI Tools
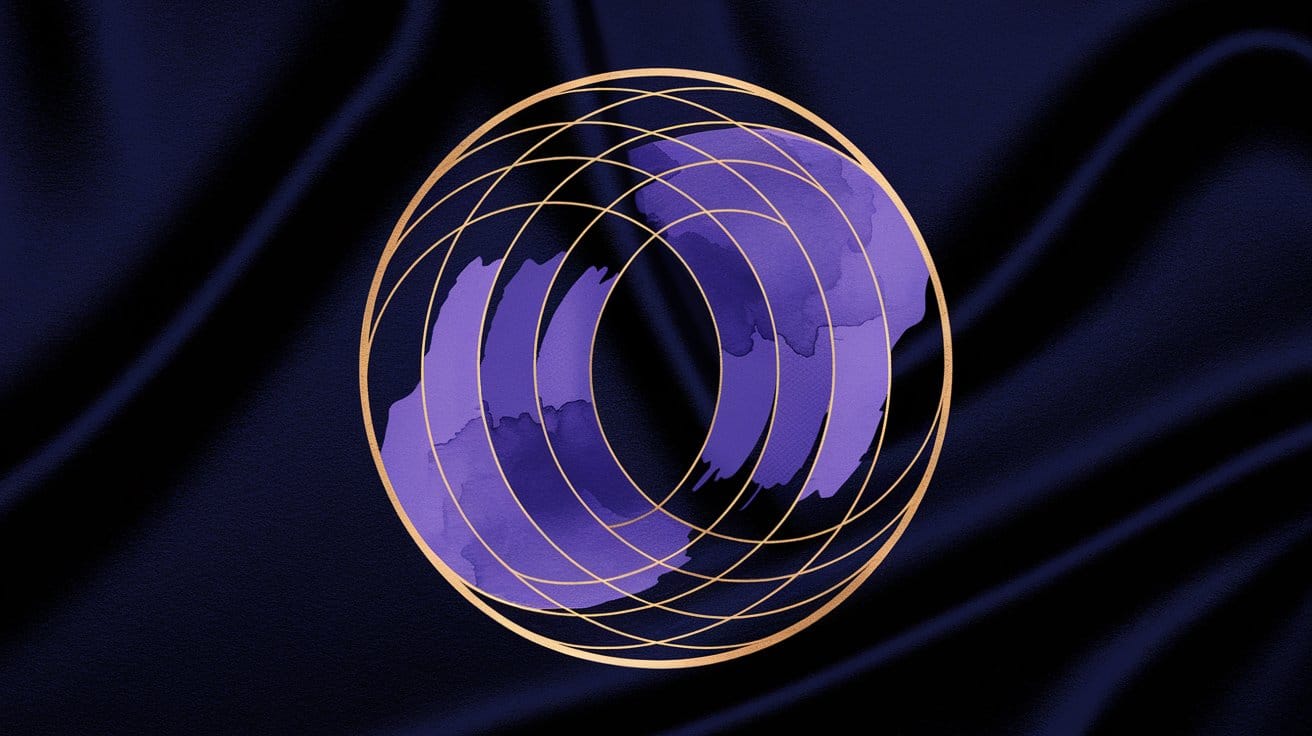
While custom AI solutions remain crucial for long-term success, there's immediate value waiting to be unlocked in today's readily available AI tools. Let's explore how to capitalize on both.
Quick Wins Through Public AI Tools
Gone are the days when AI implementation meant months of development. Tools like ChatGPT, Claude, and Gemini can revolutionize your workflows within days. The key? Start small and start now. Whether it's drafting customer communications, analyzing market reports, or streamlining documentation, these quick wins build confidence and demonstrate tangible ROI—making it easier to secure buy-in for larger AI initiatives.
These tools are your digital transformation accelerators, particularly powerful for:
- Writing and Content: From polished email drafts to compelling marketing copy
- Data Analysis: Transform raw data into actionable insights
- Code Development: Speed up debugging and documentation tasks
- Research: Accelerate market intelligence gathering and trend analysis
- Customer Service: Enhance response quality and speed
Mastering Prompt Engineering
If data is the fuel of AI, prompts are the steering wheel. Strong prompt engineering skills help your teams extract maximum value from AI tools while maintaining consistency and quality. Here's what to focus on:
Training Fundamentals:
- Prompt Design: Build expertise in crafting clear, specific prompts that get reliable results
- Context Management: Master the art of maintaining conversation flow and context
- Quality Control: Implement verification steps and best practices for consistent outputs
Building Your Prompt Library:
Create a shared repository of proven prompts for common tasks. Think templates for customer responses, report analysis, or code documentation. This standardizes output quality and saves countless hours of reinventing the wheel.
Hybrid Implementation Strategy
Smart organizations don't choose between custom AI and public tools—they leverage both. Public AI tools offer quick wins and learning opportunities, while custom solutions handle specialized needs and sensitive data.
When to Use Public AI:
- Rapid prototyping and experimentation
- General knowledge tasks
- Content creation and editing
- Initial data analysis
- Learning and skill-building
When to Go Custom:
- Handling sensitive company data
- Industry-specific applications
- Integration with proprietary systems
- High-volume, specialized tasks
- Competitive advantage areas
Remember to establish clear guidelines around data sharing, prompt standards, and security. It's about finding that sweet spot between quick wins and thoughtful development—building momentum while laying the groundwork for more sophisticated AI implementations down the road.
Would you like me to adjust anything about the tone or structure to better match the rest of your blog?
Common Pitfalls and Challenges
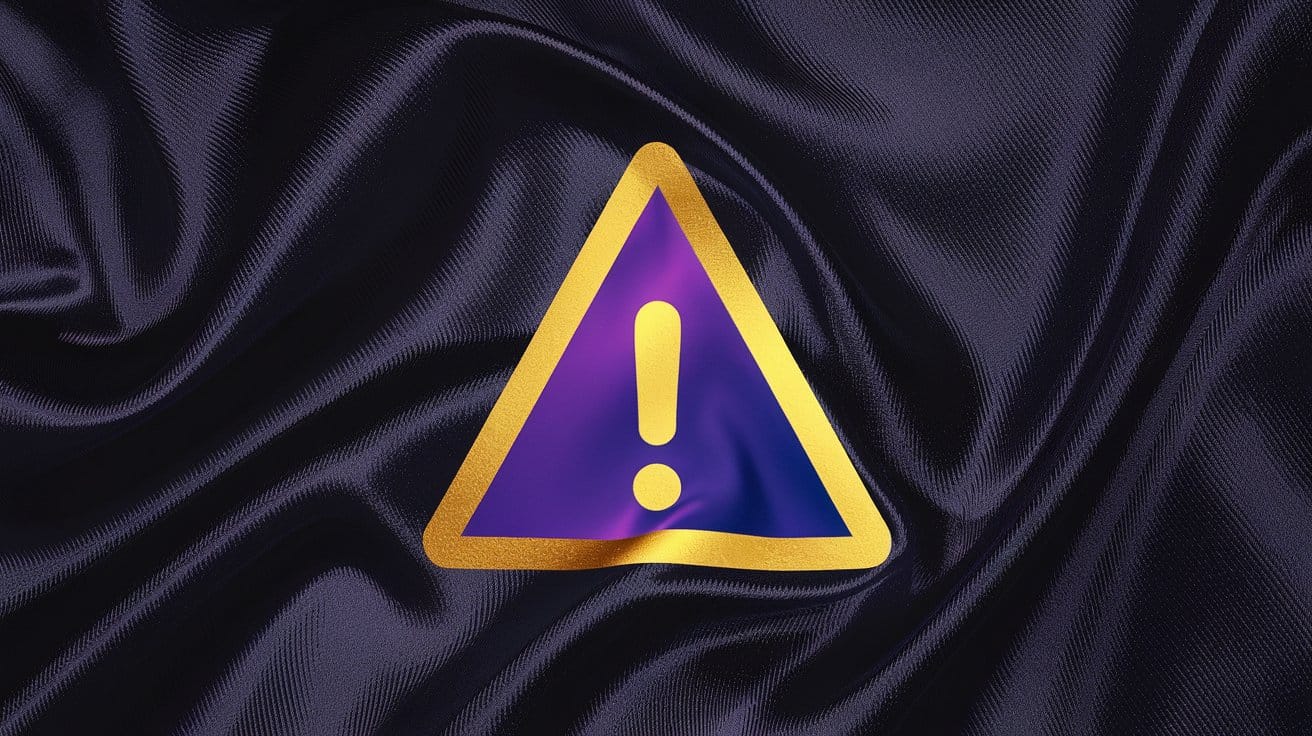
Data Readiness and Quality
If your data is scattered or messy, your AI projects could flop. Invest in solid data governance and a dedicated team or process to maintain data integrity. A fancy algorithm is useless if it’s trained on poor data.
Balancing Speed with Responsibility
Move too fast, and you risk letting bias or privacy mishaps slip through. Move too slowly, and you might miss valuable opportunities. Strike a balance: perform thorough risk assessments—checking for bias, compliance, and security—while still encouraging innovation and experimentation.
Maintaining Momentum Post-Launch
A lot of AI initiatives start strong but then fade away once the novelty wears off. Keep top execs involved, celebrate each milestone, and tie performance metrics to AI-driven improvements. Rotate employees into AI-focused roles so knowledge doesn’t sit in a single silo.
Core Takeaways
- Plan Strategically: Clearly define your AI goals, find your skill gaps, and outline a phased plan.
- Adopt a Tailored Approach: Use both in-house and external training for a balanced, effective rollout.
- Build a Supportive Culture: Communicate openly, be transparent about AI’s role, and show strong leadership endorsement.
- Measure and Refine: Track numbers and stories. Celebrate small wins, adjust as you go, and keep on iterating.
- Stay Ethical and Future-Focused: Weave in responsible AI practices and keep tabs on evolving tech and regulations.
Feeling ready? Pick a pilot project, bring the right decision-makers on board, and begin laying your AI training foundation. Document everything—wins, lessons, and areas needing improvement. Over time, keep scaling up, refine your approach, and stay curious. By embracing AI systematically and keeping an eye on the future, you’ll transform your organization into an AI powerhouse, poised to thrive in a fast-evolving world.