2025 AI Trends: The Complete Enterprise Guide & Forecast
Discover the 8 critical AI developments shaping business in 2025, from agentic AI to ROI optimization. Get practical strategies for responsible AI adoption, cost control, and competitive advantage in this comprehensive enterprise guide.
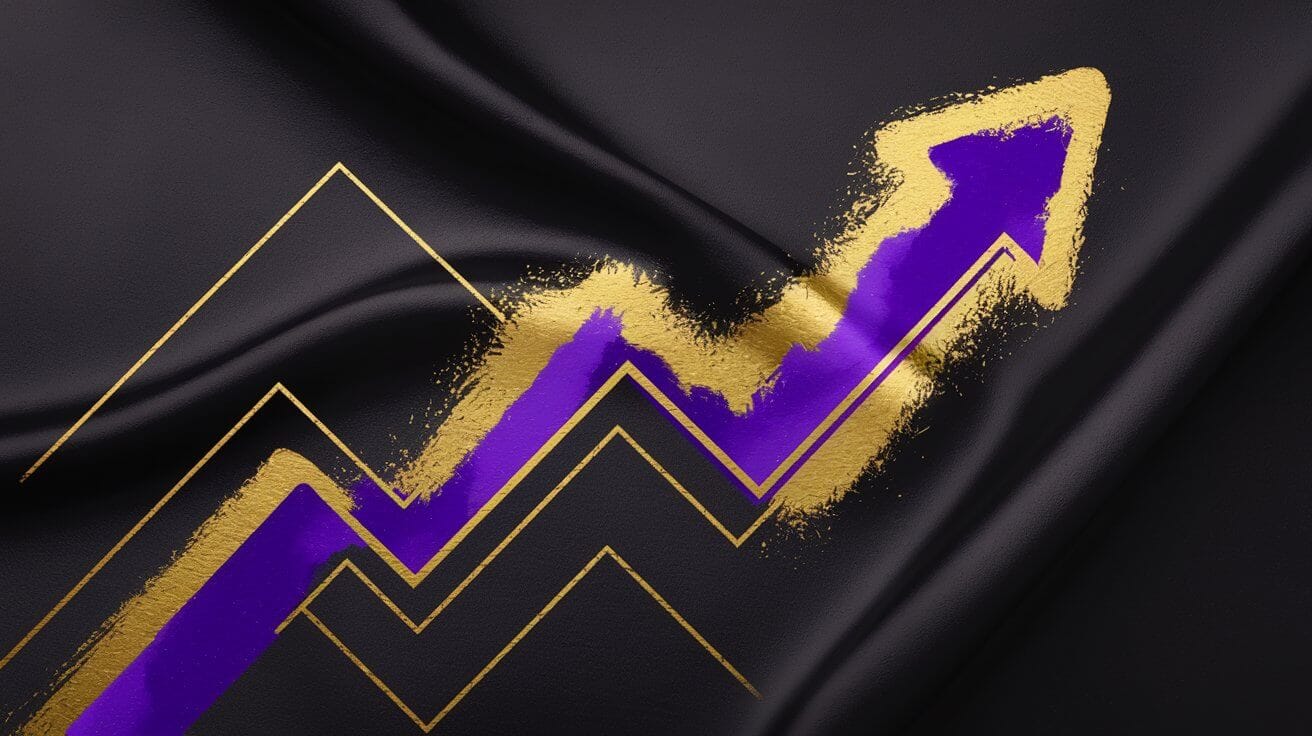
Remember when 2024 felt like a whirlwind of AI breakthroughs? Well, 2025 is shaping up to take us even further. We’re now seeing an exciting convergence of agentic AI, small language models, and stronger governance frameworks—efforts meant to drive innovation while keeping security and ethics at the forefront. If you’re mapping out an AI roadmap for the coming year, this is an ideal time to get a handle on these trends, refine your cost and compliance strategies, and ready your teams for the next wave of AI opportunities.
Below, we’ll look at the major developments shaping AI in 2025, offer key adoption considerations, and suggest practical strategies to help you stay competitive—from LLM cost optimization to industry-specific readiness checklists. Whether you’re a lean startup or a multinational enterprise, these insights can guide you in making AI moves that are responsible, robust, and ready for whatever the market demands next.
1. Agentic AI: Beyond Simple Automation
Agentic AI is generating a lot of buzz this year. It involves autonomous systems that plan, act, and even collaborate with minimal human oversight—taking us beyond the chatbot pilots we saw back in 2024. From my experience, the biggest payoff appears in customer service, IT operations, and sales automation, where agentic AI can offload repetitive tasks, freeing your teams to focus on more strategic, higher-value initiatives.
- Customer Service: Intelligent AI “agents” can handle routine queries and transactions, letting human teams handle nuanced, personalized support.
- IT Operations: Automated cloud resource provisioning and proactive monitoring help cut downtime and keep operations steady.
- Sales Automation: Agentic AI solutions can handle prospecting and follow-up so sales teams can focus on building relationships and closing deals.
However, agentic AI has its share of challenges. Real-time decision-making raises significant security and compliance questions. You’ll want robust safeguards—like strict identity management and prompt injection defenses—plus clear human accountability. As these systems expand into more complex workflows, strong oversight becomes even more crucial.
Security by Design for Agentic AI
Securing agentic AI is non-negotiable. Consider these key practices:
- Least-Privilege Access: Restrict each AI agent to only the data and tools it genuinely needs.
- Ongoing Threat Monitoring: Use intrusion detection and real-time logging to spot malicious prompts or suspicious activities.
- Agent Lifecycle Management: Consistently audit agent behaviors to ensure they still align with compliance and safety standards—even as models or workflows evolve over time.
2. Small Language Models (SLMs) and Specialized Models
Small language models (SLMs) are gaining ground in 2025, especially for mid-sized businesses looking for powerful AI without colossal infrastructure bills. They’re resource-efficient and often excel at focused tasks or specialized domains.
- Efficiency and Speed: SLMs typically demand less hardware, enabling quick text processing and cost-effective inference.
- Domain-Specific Excellence: Fine-tuned SLMs can outperform bigger models in specialized areas like financial compliance or medical triage.
- Embedded & Edge Applications: Because SLMs are smaller, they can run locally, making them ideal for scenarios with limited connectivity or strict data sovereignty rules.
That said, large-scale LLMs still have a place when the problem space is truly complex. In reality, many organizations will pair smaller, targeted SLMs for certain sub-tasks with more robust, general-purpose LLMs when needed.
3. Generative AI & Multi-Modal Use Cases
Generative AI is far from cooling off. In 2025, it’s expanding beyond text to images, audio, and even video. More companies are integrating multi-modal features into daily workflows, particularly in marketing, design, and content production.
- Marketing & Creative: Generate ad copy, design prototypes, or short video clips at scale.
- Education & Training: Provide immersive lessons that merge text, graphics, audio, and occasionally virtual lab components.
- Healthcare & Diagnostics: Combine patient imaging with medical records for a more thorough clinical assessment.
Yet these multi-modal applications also introduce concerns about copyright, misinformation, and deepfake risks. My advice? Support these capabilities with clear usage policies and a firm grasp on your training data. You’ll thank yourself later.
4. RAG (Retrieval-Augmented Generation), Data-Centric AI, and Knowledge Management
For organizations with a wealth of internal data, data-centric AI isn’t just a buzzword—it’s critical. Retrieval-augmented generation (RAG) lets models pull from proprietary databases in real time, cutting down on hallucinations and delivering context-rich responses. Combining RAG with verified data stores can make outputs both safer and more credible.
- Safer, More Accurate Queries: Verified data reduces hallucination risk.
- Domain Adaptation: RAG can be customized for specialized fields like finance or healthcare with minimal re-training.
- Knowledge Graphs & Version Control: Though indexing large repositories and maintaining prompt structures can be complex, up-to-date data pipelines ensure the most accurate results possible.
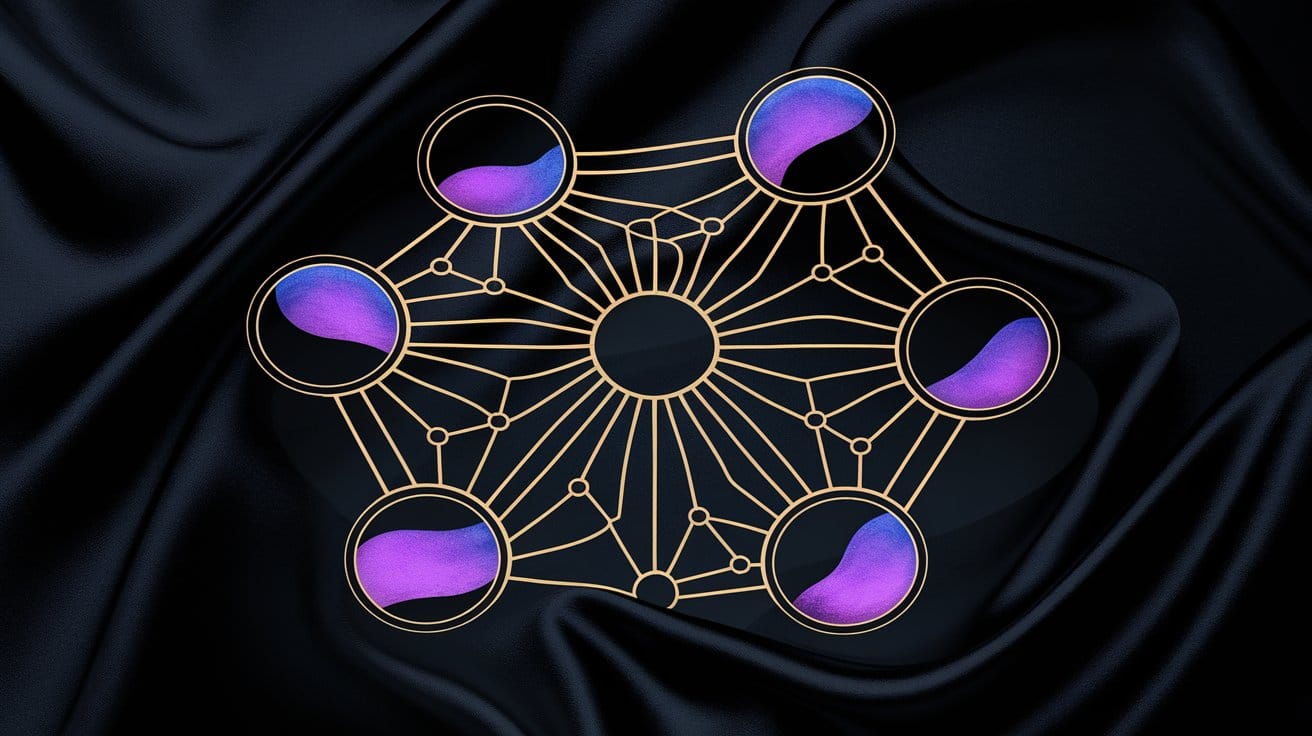
5. AI Governance, Compliance & Ethical Usage
After several headline-grabbing data breaches in 2024, governance and compliance are top priorities in 2025. We’re seeing new regulations shape up, including the EU AI Act and emerging transparency laws in various U.S. states. Last year’s breaches also accelerated demands for stronger oversight.
- Expanded Regulations: The EU AI Act and other frameworks set strict rules on privacy, disclosure, and ethical design.
- Industry-Specific Rules: Fields like finance, healthcare, and education have unique standards (SEC guidelines, HIPAA, etc.).
- More Audits & Vendor Checks: Customers, especially in B2B and government sectors, increasingly demand proof that AI systems meet security and compliance benchmarks.
In-Depth Governance/Compliance Roadmap
Not all organizations share the same governance needs, so there isn’t a universal formula. A startup may stick to simpler policies, whereas a large enterprise might assemble a full ethics board. Here’s a quick tiered checklist:
- Startups: Basic encryption, minimal data collection, and a bit of interpretability.
- Mid-Sized Orgs: Formal AI usage policies, a designated AI officer or committee, and sector-specific compliance (GDPR, PCI-DSS, etc.).
- Enterprises: Comprehensive AI governance boards, risk modeling, ethics committees, ongoing scenario testing, and regular external audits.
6. LLM Cost Optimization & Infrastructure Planning
Large generative models can deliver remarkable capabilities, but they’re also notorious for hefty compute costs. In 2025, cost optimization is a major topic as businesses aim to scale without draining their budgets.
- Quantization & Pruning: Reduce model size to achieve faster, cheaper inference.
- Pay-Per-Token vs. Subscription: Light usage might favor pay-per-token billing, while heavy usage could justify a monthly or annual plan.
- Hybrid Deployment: For day-to-day workloads, on-prem HPC clusters can be economical, with cloud inference for spikes.
- Caching & Reuse: Storing frequently used embeddings or responses can cut down on repeated computations.
ROI Frameworks for Mid-Sized Enterprises
Many mid-sized organizations see a quick return on AI investments, often within 3–6 months, especially for functions like customer service triage or marketing copy generation. Try this approach:
- Map Key Use Cases: Identify processes that would benefit most from LLM or agentic AI.
- Estimate Cost Scenarios: Compare pay-per-token, subscription, or on-prem hosting models.
- Pilot & Measure: Start small to gather real usage metrics.
- Refine & Iterate: Shift to smaller models or adjust prompts for efficiency.
- Calculate Payback Period: Link actual cost savings or new revenue directly to your AI spend.
7. Industry-Focused AI & Workforce Strategies
AI’s impact will be felt in every sector, but each industry has distinct requirements. In 2025, we’ll see more customized AI solutions:
- Finance: Real-time fraud detection, sophisticated credit models, and multi-agent risk simulations.
- Healthcare: Automated triage, remote patient monitoring, and specialized SLMs to assist clinical decision-making.
- Retail: Demand forecasting, hyper-personalization, and AI-driven supply chain optimization.
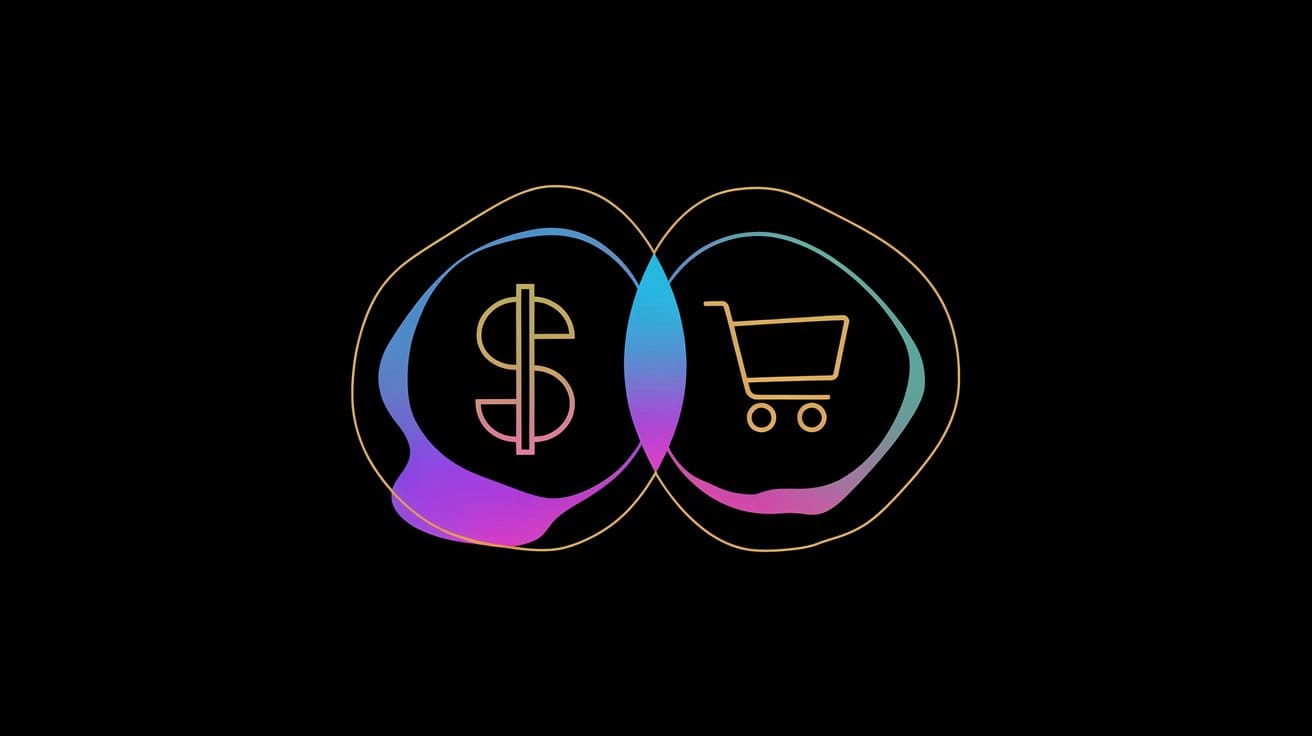
Sector-Specific Readiness Checklists
Each vertical faces its own regulatory challenges, from SEC rules in finance to HIPAA in healthcare. Having a customized checklist for your industry can help you dodge common mistakes and accelerate AI adoption.
Workforce Upskilling & AI Talent
As AI extends its reach, skill gaps inevitably widen. Plenty of teams still need to learn about prompt engineering, data analytics, or AI ethics. In my experience, a balanced approach—combining internal upskilling, external expertise, and collaborative team-building—can bridge these gaps effectively, ensuring that AI deployments are both technically and practically sound.
8. Sustainability & Ethical AI by Design
The rise of LLMs and agentic AI underscores the need for responsible data practices and environmental stewardship. In other words, don’t tack on sustainability and ethics at the end—build them into your design from day one.
- Green AI Practices: Smaller, more efficient models, renewable-powered data centers, and continuous tracking of carbon footprints.
- Bias Mitigation: Regularly audit training data, especially for consumer-facing or sensitive applications.
- Explainability & Transparency: Favor interpretable model architectures and clearly inform users when AI is in play.
- Global Framework Alignment: Adhere to guidelines from groups like OECD or UNESCO, and consider adding multi-stakeholder oversight boards.
Final Takeaways: Building AI Future-Readiness
As we head into 2025, it’s evident that agentic AI, small language models, RAG systems, and cost optimization are set to dominate discussions. But remember: security, compliance, and ethical responsibility need to be baked into your AI initiatives from the ground up.
No matter your organizational size, here are five must-do steps:
- Evaluate Resource Needs: Pick the right type of AI (SLMs vs. large LLMs) based on usage and ROI.
- Adopt Governance Frameworks: Tailor compliance guidelines to your specific industry and organizational scale.
- Secure from Day One: Protect agentic AI with strong identity management, data privacy measures, and threat monitoring.
- Upskill Continuously: Close skill gaps via training, external experts, and cross-functional teams.
- Embed Sustainability & Ethics: Reduce bias, stay transparent about where and how AI is used, etc.
By balancing innovation, cost control, and responsible governance, you’ll be in a prime position to ride AI’s continued evolution throughout 2025—turning forecasts into tangible results for your business and the communities it serves.